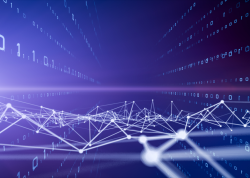
This blog is provided by the IPR Measurement Commission.
Accurate and timely media analysis is crucial to shaping public relations strategies and measuring audience impact. Artificial intelligence (AI) can efficiently sift through vast amounts of content in minutes, often reducing the time to identify trends and sentiment from hours or days to mere minutes (Whitaker, 2017).
However, as organizations increasingly adopt AI for data processing and insights, it is essential to identify best practices around when to use AI and when to rely on human expertise. Human insight is often irreplaceable when analyzing nuanced topics or datasets. While technologies such as automation and machine learning have been successfully used in PR, an industry-wide hesitation exists around large-scale AI implementation due to accuracy gaps and transparency issues.
After leading media measurement teams for 15-plus years, I’ve learned that AI usage doesn’t have to be an either-or approach. Strategically combining the strengths of AI with the critical thinking and creativity of PR professionals allows organizations to accelerate and enhance media analysis efforts, leading to more informed decision-making and impactful communication strategies.
Humans Where Humans Make Sense; Machines Where Machines Make Sense
Researchers found that human sentiment coding had an average accuracy rate of 85% compared to 59% for AI (van Atteveldt et al., 2021). It is important to note that while human coding accuracy ranks higher than the machine, AI outranks humans in terms of efficiency. An exploration of AI’s use in coding practices shows a 40% reduction in analysis time with AI (Kakhiani, 2024), and industry reviews recognize the superpower of AI to work 100 times faster than human coders (Diamandis, 2024; Kaoukji, 2023).
So, what’s the lesson? The key is to use humans where humans make sense and machines where machines make sense. Different factors and contexts should be considered:
— Humans make the most sense when you have more time, when the data volume is more manageable, when accuracy is crucial, when results will inform senior-level decision-making, and when topics are more complex or nuanced.
— When a fast turnaround is of the essence — such as crisis response — or when you’re using massive datasets (or when topics are more clear-cut), AI with human supervision is likely a better option.
It’s also imperative for organizations to enact AI usage and disclosure policies and introduce greater transparency into the AI models used, to maintain and build trust with audiences.
“This means higher data standards, greater transparency and documentation of AI systems, measurement and auditing of its functions (and model performance), and enabling human oversight and ongoing monitoring,” explains Converseon founder and IPR Measurement Commission member Rob Key. “In the near future, it is likely that almost every leading organization will have a form of AI policy in place that will adhere closely to these standards.”
Human vs. Machine: Best Practices and Factors to Consider
In most cases, AI and humans should be used side-by-side. Organizations should defer to human-in-the-loop AI models, which include human input in the model’s training and outputs and consider a range of factors when deciding whether to deploy machines or human resources. Here are the most impactful:
1.) Timing and Speed: AI can process data much faster than humans, making it ideal for time-sensitive analyses.
2.) Data Volume: AI excels at identifying patterns and trends in large datasets that may be missed by human analysts.
— This makes machines ideal for tasks such as tracking mentions or identifying trends across wide datasets. But in complex industries such as healthcare or financial services, understanding the implications of regulations, policies, or industry-specific jargon is critical and is likely better suited to a human.
3.) Accuracy: AI often struggles with nuanced interpretations, while human coders can apply context and critical thinking in analyses where subtlety matters. Human coders can also contextualize and verify automated results.
— When performing sentiment analysis, automated tools struggle with nuances like sarcasm, cultural context, and double meanings.
4.) Audience: AI might lack the sensitivity needed for certain audiences. If the analysis needs to resonate deeply with a specific demographic or requires a nuanced contextual understanding, human coders may be a better fit.
5.) Decision Impact: If the results will drive significant business decisions, the depth of understanding that human analysts provide might be more appropriate. The stakes involved can justify the added time and resources.
6.) Topic Complexity: AI excels in straightforward, data-driven analyses. For intricate or abstract subjects that require deep understanding or emotional intelligence, human analysts may be more effective.
— Human curation is vital when assessing the credibility and impact of sources. Media measurement is more than just counting mentions or clicks: It’s about understanding who is speaking, their level of influence, and their quality of engagement.
From my experience and available research, I consider it best practice to use machines for initial data collection, aggregation, and basic sentiment analysis, and incorporate human analysis for contextual understanding, sentiment refinement, and evaluating the importance of key opinion leaders or sources. It’s also important to regularly audit automated tools for accuracy.
Conclusion
Integrating human expertise with automation is vital to delivering comprehensive and reliable media measurement. Media analysis companies can combine trusted human analysis and advanced AI capabilities to provide quality and timely results. However, organizations must also be transparent in their use of AI to support informed output consumption.
Indeed, the rising prioritization of trusted AI — ensuring that AI systems are transparent, reliable, and ethically sound — means organizations must employ ethical guidelines regarding the usage of AI. By building trust in AI technologies and supplementing AI’s efficiency with human insight, organizations can harness the technology’s full potential while safeguarding against biases and inaccuracies, ultimately leading to more informed and impactful outcomes in media analysis.
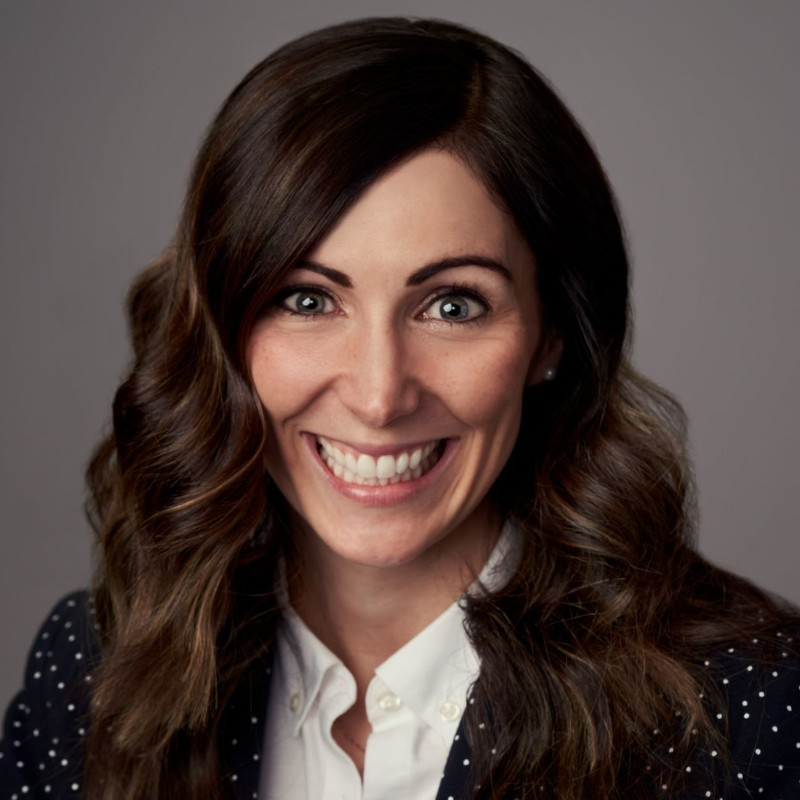
For more than 15 years, Angela Dwyer has balanced human expertise and automation in PR measurement to help brands prove value and improve communications performance. Angela is Head of Insights at Fullintel and director of the IPR Measurement Commission. She has developed measurement systems, expanded global companies, and designed science-backed metrics.